Fig. 16.1
Predictors of global function in bipolar disorder
16.3.2 Grey Matter
Grey matter changes in BD are generally subtle as their average effect size is 0.5 or less (Kempton et al. 2008). There is little evidence for global grey matter abnormalities especially in first-episode patients (Vita et al. 2009). Regional grey matter volume reductions have been consistently reported in BD patients compared to healthy individuals in the VPFC, insula and ACC (Bora et al. 2010; Ellison-Wright and Bullmore 2010; Selvaraj et al. 2012). Despite initial reports implicating the amygdala, meta-analyses of sMRI data from voxel brain morphology (VBM) studies in adult BD patients have not found consistent alterations in this structure. Similarly, meta-analyses of region-of-interest (ROI) studies report minimal differences in amygdala volume between adult BD patients and healthy individuals (Hedges’ g −0.04 to −0.07) (Kempton et al. 2008). However, amygdala volume reduction has emerged as a consistent finding in paediatric BD (Terry et al. 2009). Evidence from longitudinal studies indicate that the smaller size of the amygdala early in life in BD may be accounted for by delayed or abnormal developmental trajectories in patients compared to healthy youth (Bitter et al. 2011).
Grey matter reductions in the VPFC and the ACC represent a robust correlate of disease expression in BD and are present regardless the age of onset. Studies that have examined the relevance of these findings to other disorders have reported overlap but also differences. Grey matter volume reductions in SZ are generally more extensive and more severe, but there is substantial overlap between the two disorders in the insula and ACC (Ellison-Wright and Bullmore 2010). Patients with SZ also appear to have greater ventricular enlargement and smaller amygdala volumes than those with BD (Arnone et al. 2009). A substantial overlap has also been found in brain structural changes in BD and MDD (Kempton et al. 2011). However, MDD patients may have smaller hippocampal and basal ganglia volume and larger corpus callosum areas compared to BD patients (Kempton et al. 2011).
16.4 Brain Function in BD
16.4.1 Resting State Studies
Functional magnetic resonance imaging (fMRI) techniques have been adapted to allow examination of resting state data and subsequent connectivity analyses of resting state neural networks. The first report was from Anand and colleagues (2009) who found decreased connectivity between the pregenual ACC and the thalamus, basal ganglia and amygdala when comparing resting state data from unmedicated symptomatic (manic = 6, depressed = 5) BD patients to that from healthy individuals. Chepenik and colleagues (2010) focused on VMPFC connectivity based on resting state data from 15 BD patients with variable symptomatology compared to 10 healthy individuals. Patients had reduced negative connectivity between the VMPFC and the amygdala and increased positive connectivity between the VMFC and the ipsilateral VLPFC and ACC. These findings were largely replicated by Chai et al. (2011) in 14 acutely manic and psychotic BD patients. In contrast to healthy individuals, patients showed positive VMPFC-amygdala and VMPFC-VLPFC connectivity. Two further studies have established that abnormal VPFC-amygdala connectivity persists in euthymic BD patients (Anticevic et al. 2013; Torrisi et al. 2013) while a further study confirmed the relevance of these connectivity finding to disease expression for BD as opposed to SZ (Mamah et al. 2013; Meda et al. 2012).
16.4.2 Activation Studies
Functional neuroimaging studies in BD have employed a variety of activation paradigms. Three recent quantitative meta-analyses have usefully summarised the literature in terms of the neural regions implicated across paradigms (Chen et al. 2011) and with regard to specificity compared to MDD (Delvecchio et al. 2012) and SZ (Delvecchio et al. 2013). Chen and colleagues reviewed 65 fMRI studies comparing BD patients to healthy individuals and categorised tasks into cognitive and emotional based on the absence or presence of emotive content. During cognitive task performance, BD patients showed abnormally decreased activation in the VLPFC, the putamen and lingual gyrus. Abnormally decreased activation in the VLPFC in patients was also present during emotional tasks where additionally patients demonstrated abnormally increased engagement in the parahippocampal gyrus extending to the amygdala and in the putamen and pallidum. Delvecchio et al. (2012) further demonstrated that increased activation of the parahippocampal gyrus/amygdala during emotional tasks was a shared feature of BD and MDD. However, diagnosis-specific differences were also observed as decreased VLPFC engagement and increased responsiveness in the thalamus and basal ganglia were associated with BD and not MDD. Comparison between BD and SZ during emotional processing tasks revealed significant diagnostic differences; SZ was associated with underactivation both in medial temporal and in prefrontal regions but greater engagement in posterior associative visual cortices (Delvecchio et al. 2013). This finding suggests that the origin of emotional processing abnormalities in BD probably relates to dysfunctional emotional regulation while abnormal visual integration may be the core underlying deficit in SZ.
16.5 Key Moderating Factors
Sex does not appear to have a major moderator role in brain morphology in BD (Jogia et al. 2012). Age and duration of illness are highly collinear. As there is a paucity of longitudinal studies in BD, the effects of these two factors cannot be easily disentangled. Sarnicola et al. (2009) did not find differential age-related changes in brain morphology in BD patients and healthy individuals over an age span of 40 years. However, multi-episode (as opposed to first episode) BD patients may have smaller VPFC and larger striatum and amygdala (Bora et al. 2010). The latter observations may reflect chronic exposure to medication and particularly antipsychotics and lithium. The association between antipsychotics and increased striatal volume is well replicated and is considered to reflect diagnosis-independent microstructural changes in response to long-term reduction in dopamine neurotransmission (Navari and Dazzan 2009). Moore et al. (2000) were the first to report that treatment with lithium increased global grey matter volume in BD patients. This observation has been robustly replicated with regard to global (Bearden et al. 2007; Lyoo et al. 2010) and regional grey matter volume increases particularly in the amygdala, rostral ACC and PFC (Bearden et al. 2007; Germaná et al. 2010; Moore et al. 2009). This brain volume “response” to lithium is of therapeutic significance as it has been linked to symptomatic improvement (Lyoo et al. 2010; Moore et al. 2009). Although the precise mechanism underlying lithium-induced brain structural changes in BD remains to be determined, the drug’s primary target, glycogen synthase kinase-3, is involved in pathways with neurotrophic, neurogenetic and neuroprotective functions (Machado-Vieira et al. 2009). Clinical symptoms at the time of scanning may also impact neuroimaging results. This is mostly the case for functional rather than anatomical studies. In general, acute symptoms, either depressive or manic, appear to increase fMRI signal in subcortical regions (hippocampus, amygdala) and reduce PFC engagement (Chen et al. 2011; Delvecchio et al. 2013).
16.6 Translational Potential of Neuroimaging for BD
The findings described above demonstrate the contribution of neuroimaging to our understanding of the neural correlates in BD. However, their impact on clinical practice has been negligible. This is primarily because conventional data analysis computes differences between groups either within predefined anatomical regions of interest (ROI) or throughout the brain using univariate mass analyses such as voxel-based morphometry (VBM). Both types of analyses are not able to differentiate between groups where mean differences are small to moderate. The ROI approach is statistically more powerful but restricted to regions that can be reliably anatomically defined. VBM requires correction for multiple comparisons that limits its statistical power to detect subtle changes. Additionally, neither approach utilises information about the spatial distribution of case-control differences. Current research interest is focusing on maximising the translational value of neuroimaging by developing new computational tools for data analysis that could assist with the clinical evaluation of patients.
Calhoun and colleagues (2008) used independent component analysis to extract mode images of brain activity during resting state and during performance on a selective attention task in patients with BD or SZ and healthy individuals. A multistage classification algorithm was then applied to concatenate each participant’s mode images into a single image. A mean image was computed for each group, and the Euclidean distance between an individual’s brain image and each group’s images was computed. Using this approach, the authors were able to classify participants according to diagnostic group with an average sensitivity of 90 % and specificity of 95 %.
Another computational approach involves the use of supervised machine learning algorithms to predict diagnostic classification. Costafreda and colleagues (2011) applied support vector machine (SVM) to functional imaging data derived from patients with BD or SZ and healthy individuals while performing a verbal fluency task. Classification accuracy for SZ was 92 % (91 % sensitivity and 92 % specificity) and for BD 79 % (56 % sensitivity and 89 % specificity); misclassification occurred mostly because of the wrong categorization of BD patients as healthy individuals. Rocha-Rego and colleagues (2013) applied Gaussian Process Classifiers (GPCs) to sMRI data to evaluate the feasibility of using pattern recognition techniques for the diagnostic differentiation of patients with BD from healthy individuals. They chose to focus on sMRI because of its high acceptability by patients and wide availability in clinical settings. GPCs represent a significant advance as they combine equivalent predictive performance to SVM with the additional benefit of probabilistic classification (Marquand et al. 2010). Grey and white matter classifiers correctly assigned patients to the appropriate diagnostic category with respective accuracy levels of 73 % (sensitivity 69 %, specificity 77 %) and 69 % (sensitivity 69 %, specificity 69 %). Grey matter discriminative clusters were localised within cortical and subcortical structures implicated in BD, including the VPFC, ACC, parahippocampal gyrus, insula, thalamus and striatum (Fig. 16.2a). White matter discriminative clusters were identified mainly within the cingulum, occipital regions and the genu of the corpus callosum (Fig. 16.2b).
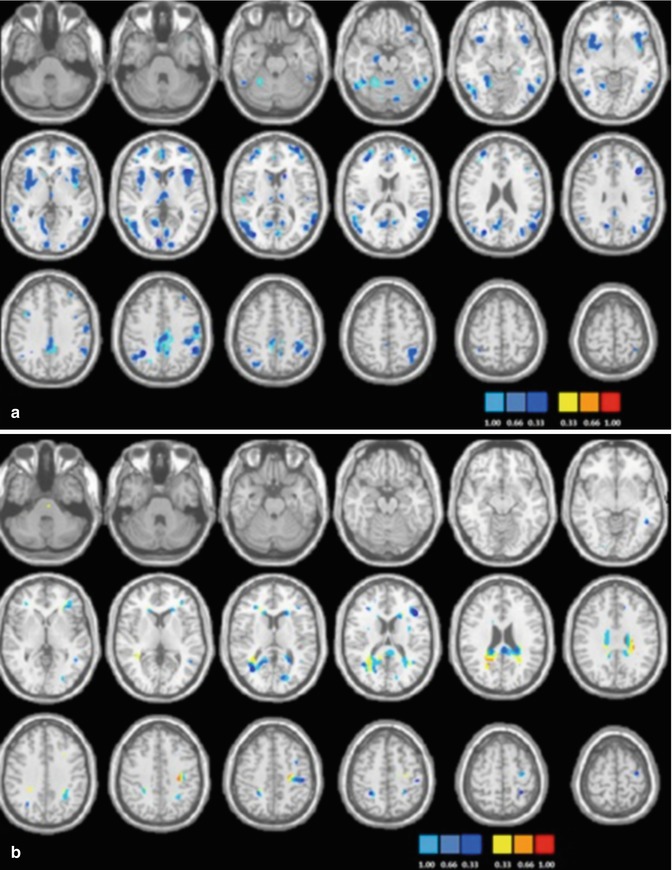
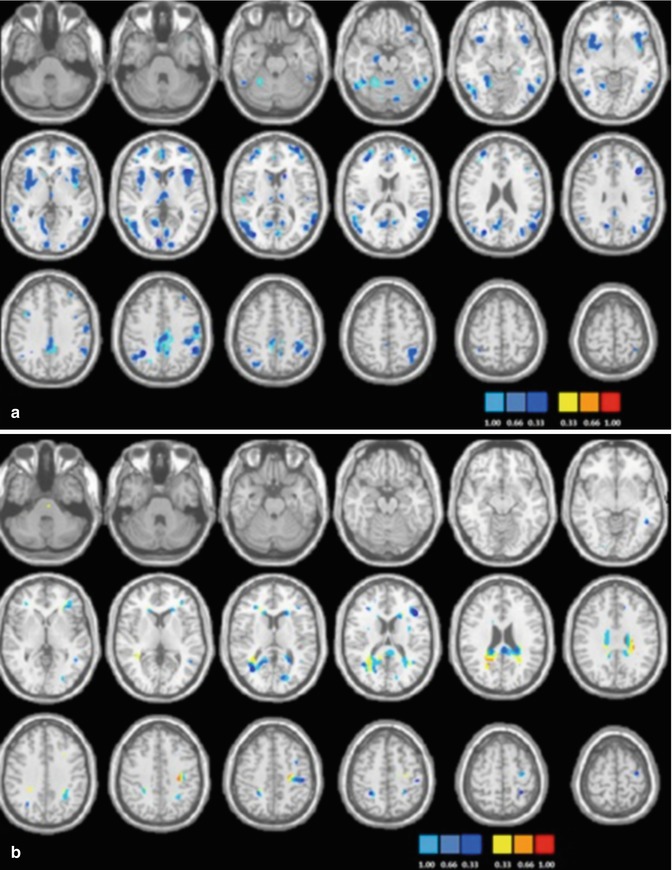
Fig. 16.2
Discrimination maps for grey matter (a) and white matter (b) classification between patients with bipolar disorder and healthy individuals
16.7 Concluding Remarks
This chapter summarised the effect of disease expression for BD on brain structure and function. There are four key messages. First, the data demonstrate the central role of VPFC dysfunction in BD. Postmortem studies in BD report neuropathological abnormalities in the VPFC leading to regional reductions in the number and density of pyramidal cells and PV interneurons (Cotter et al. 2005; Pantazopoulos et al. 2007). The mechanisms involved are not established, but multiple lines of evidence implicate reduced expression of neurotrophins (Berk et al. 2011), abnormalities in oxidative energy generation (Berk et al. 2011) and mitochondrial dysfunction resulting in altered Ca+2 regulation and PV-interneuron reduction (Berk et al. 2011; Powell et al. 2012). Second, the evidence suggests that medial temporal lobe regions (amygdala/parahippocampal gyrus), although not structurally compromised, are functionally abnormal in terms of neural responsiveness and connectivity, both consistent with loss of regulatory input. Third, additional abnormalities are present in subcortical regions, particularly the insula, thalamus and basal ganglia, suggesting that mechanisms relating to BD impact on the representation and awareness of feelings toward stimuli or events (insula) (Craig 2011), directing and maintaining attention toward emotional stimuli (thalamus) (Pessoa and Adolphs 2010), and on response selection (basal ganglia) (Robins 2007). Fourth, increased computational sophistication may allow in the near future the use of neuroimaging as a clinical evaluation tool. The data summarised here also highlight a major knowledge gap. That is, although we are able to define the “biological phenotype” of BD in increasing detail and precision, we are still behind in delineating the pathophysiological mechanisms that contribute to this phenotype.
References
American Psychiatric Association (1994) Diagnostic and statistical manual of mental disorders: DSM-IV. American Psychiatric Press, Washington, DC
Anand A, Li Y, Wang Y, Lowe MJ et al (2009) Resting state corticolimbic connectivity abnormalities in unmedicated bipolar disorder and unipolar depression. Psychiatry Res 171:189–198PubMedCentralPubMedCrossRef
Anticevic A, Brumbaugh MS, Winkler AM et al (2013) Global prefrontal and fronto-amygdala dysconnectivity in bipolar I disorder with psychosis history. Biol Psychiatry 73:565–573PubMedCentralPubMedCrossRef
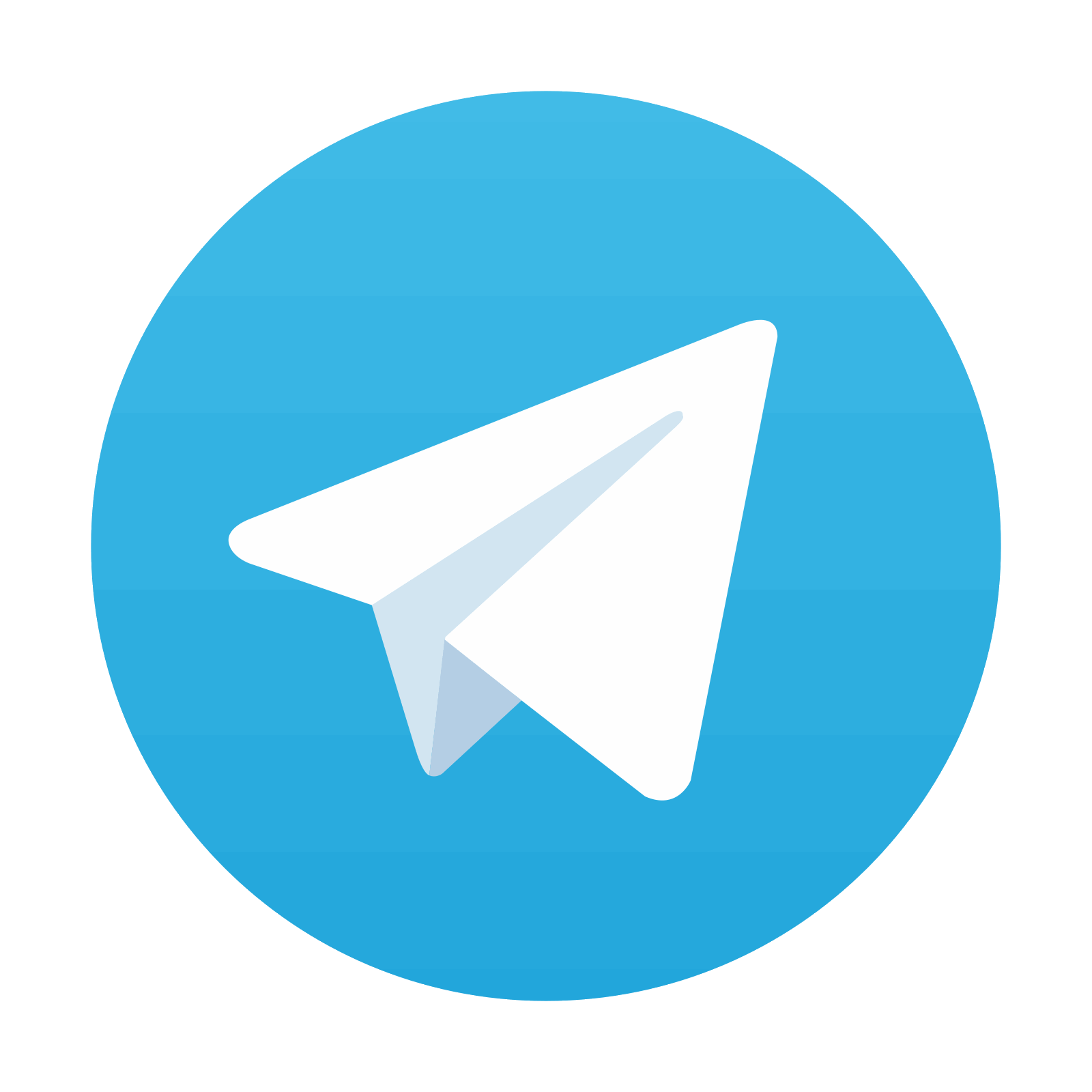
Stay updated, free articles. Join our Telegram channel

Full access? Get Clinical Tree
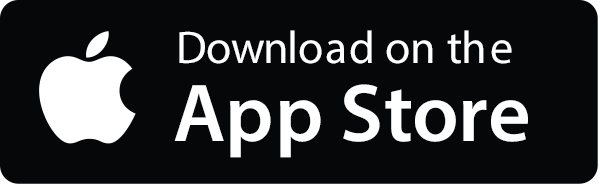
